Institute for Model-based Qualification and Certification of Additive Manufacturing
The Institute for Model-based Qualification and Certification of Additive Manufacturing (IMQCAM) is a project of the NASA Space Technology Research Institute (STRI) led by Carnegie Mellon University and Johns Hopkins University. It aims to deliver a Digital Twin (DT) platform that will ensure the Quality and Certification (Q&C) of Metal Additive Manufacturing (MAM) processes and products.
Tony Rollett, professor of materials science and engineering at Carnegie Mellon, and Somnath Ghosh, professor of civil and systems engineering at Johns Hopkins University, are co-directors of this multi-institutional team.
Digital Twin Technology for Additive Manufacturing Certification
The digital twin technology developed by the IMQCAM will consist of a powerful set of integrated computational models and simulation tools. These tools can promote a holistic, cradle-to-grave model-based approach to unraveling complex subtleties in the materials-processes-structure-property performance and life linkages for metal additive manufacturing. Such an ambitious goal can only be achieved through an integrated team effort under the aegis of the Institute.
The unique platform will draw strength from the innovation and integration of modules involving Experimental and Computational Mechanics & Materials, coupling Integrated Computational Materials Engineering (ICME), Multiscale Modeling and Design, Uncertainty Quantification (UQ), Verification and Validation (V&V), and Data Processing & Software Integration.
The digital twin will drastically reduce the testing burden, thereby reducing costs for Q&C of MAM, with increased confidence in component performance and life.
The digital twin will attain a high level of mission readiness, having been validated against ground truth experimental data from multiple sources, verified against physical mechanisms, and subject to rigorous UQ/V&V protocols.
The IMQCAM-generated digital twin will serve as a certifiable surrogate for a printed physical asset, transcending processing to component performance and life.
The problem-solving approaches and pervasive data curation will attract partners across the MAM community, who can contribute data and models and, in return, benefit from access to the DT platform as it develops. We envision the DT platform as a game-changer in model development and Q&C process that will enable the MAM community to adopt an increasingly model-centric approach to improving materials selection/design, processing conditions, and properties and ultimately improving part performance.
Our definition of the digital twin is a suite of integrated multi-physics, multi-scale computational models manifesting the cradle-to-grave processes in additive manufacturing that accepts as input feedstock information, printing process information, material microstructure to part response mapping information, part design, and service requirements. It produces output predictions of location-specific fatigue life in the form of a spectrum of life values. Its validated process-microstructure-properties-response and life models make it applicable to various materials and AM processes.
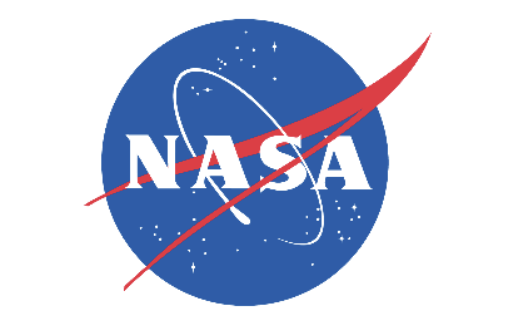